- DiggerInsights
- Posts
- Deep Dive: Demystifying LLM Technology Volume 4
Deep Dive: Demystifying LLM Technology Volume 4
LLMs Advancements and the Future of Language Technology

Mornin’ miners⛏️,
Happy Tuesday!
Digger Insights is your easy-to-read daily digest about tech. We gather tech insights that help you gain a competitive advantage!
Let’s get to it!
Today’s Deep Dive: 🤖Demystifying LLM Technology Volume 4 - LLMs Advancements and the Future of Language Technology💻

Demystifying LLM Technology Volume 4 - LLMs Advancements and the Future of Language Technology
We have gone through 3 whole volumes of the Demystifying LLM Technology Deep Dive, first discussing the basics and fundamentals of LLMs to understand their purpose, their components, and their successful applications across various industries. We then delved into the step-by-step process and challenges of LLM training as well as the impact of said training.
Last week, we explored ways for enterprises and innovators to utilize LLMs, whether in the form of chatbots, personalized systems, or voice assistants and virtual agents. This time, in the fourth and final volume of the Demystifying LLM series, we’ll learn more about how LLM has advanced over the years, in addition to the potential future of this revolutionary language technology.
LLM Research and Models
We now know that the knowledge LLMs obtain and understand depends on the data sources they are trained on. Whether trained using books or articles found on the internet, every large language model may have different skills and abilities with which they are deemed “experts.” Their different training styles are what make them generate different details in answers, making trying out various LLM applications quite exciting.
When comparing the two chatbots, Google’s Bard and OpenAI’s ChatGPT, for example, the former, developed using Google’s LLM LaMDA, can pull real-time updated information from the internet, making Bard the perfect one to use when you need the latest information. The latter, on the other hand, used training data up to 2021, so you might have difficulty trying to get responses related to the latest news and research.
The differences between various models are not limited to up-to-dateness. Some models are superior in natural language inference and question answering, while others may be miles ahead in content generation, summarization, or sentiment analysis. All of this depends on parameters, too.
These parameters shape the AI’s understanding of language and influence how the model behaves. Most companies behind LLMs are open about their model’s parameters, like LaMDA with 137 billion, GPT-3 with 175 billion, and BLOOM with 176 billion. Canada-based organization Towards Data Science published its research comparing various popular LLMs’ capabilities and differentiators.
LLMs started out as “simple” programs that can simulate human conversation, mostly used to generate creative content or just for fun. Now, with the tech constantly evolving and new LLMs being created every day, its applications have become endless. However, their development hasn’t stopped at just text.
Multimodal LLMs
Multimodal LLMs are models whose performance isn’t merely text-based. Multimodal LLMs combine text with other kinds of information like images, videos, and audio. These models essentially integrate vision and language and can accomplish tasks like describing images and videos, classifying images, or processing texts that are embedded in images.
By having the ability to not only understand the human language but also the meaning of visual media, these models can now provide visual-based responses and improve in language-related tasks. It’s quite exciting to think about the potential use cases for models such as these, especially for businesses. They can be integrated into security cameras used to monitor traffic violations and potential accidents or be utilized in e-commerce and marketplace services.
Microsoft introduced its multimodal model, KOSMOS-1, in a paper titled “Language Is Not All You Need: Aligning Perception with Language Models.” KOSMOS-1 is demonstrated to perform nonverbal reasoning and transfer knowledge across different modalities.
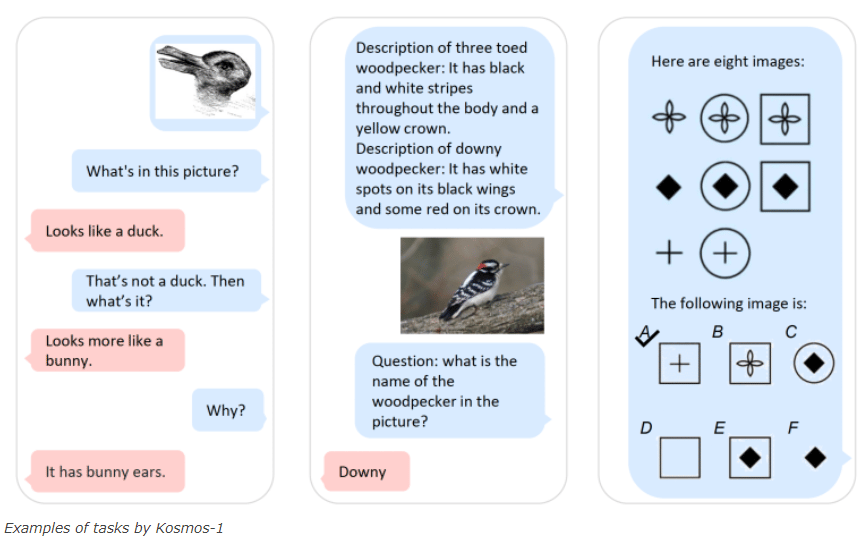
Photo Courtesy of Microsoft
Multimodality is deeply integrated into the human body, and embedded into our nervous system. We spend our whole lives shaping unconscious perceptions and abilities by experiencing the world. With mimicking human behavior and thoughts being one of LLMs’ main goals, it might take a long time and a ton of more training for them to keep up.
Cross-Lingual and Multilingual LLM Applications
Multilingual large language models, often also referred to as MLLMS, learn information in and for multiple languages simultaneously. This gives them the ability to detect and learn universal patterns and cultures, functioning in multiple languages. By being trained on a dataset that includes examples from multiple languages, however, they might not always be able to perform as high in performance compared to models trained on a single language.
GPT is amongst one of the few LLMs that are multilingual. With its language-processing feature, the model is able to detect, translate, and respond in over 95 languages by identifying the language used in a user’s question or prompt. BLOOM, an open-source model created by SambaNova and Together, is trained in 46 languages, making it the largest multilingual open model at the moment. I tested BLOOMChat by conversing with the model in Indonesian.

Photo Courtesy of BLOOM and huggingface
Cross-lingual LLMs are also models that can handle multiple languages, similar to MLLMs. What differentiates the two is while MLLMs are trained on multiple languages simultaneously, cross-lingual models are trained on one language and then adapted to other languages using transfer learning techniques, a technique we learned in Volume 2.
By being trained on a dataset that includes examples from one language, these models may be able to achieve high performance in multiple languages, but more data and computational resources would have to be used so the models can adapt to new languages.
OpenAI
As previously mentioned, GPT is one of the few MLLMs out there, but this hasn’t always been the case. Being one of the largest and most known models, its creator, OpenAI, has massively contributed to the advancements of LLMs while still continuously developing their products as well.
Back when OpenAI was first created in 2015, the team’s mission was to develop AI tools to empower people. They released the very first version of GPT (Generative Pre-trained Transformer) in 2018, which was already trained on vast amounts of data, giving it the ability to generate human-like text by mimicking the human brain’s structure and function, which we know as deep learning.
Though GPT was already deemed quite powerful, OpenAI released GPT-2 in 2019, a model with 1.5 billion parameters. The model was only able to operate in English, but it improved significantly in content creation, language translation, and code generation compared to its predecessor.
Before releasing the paid, updated version of GPT, that is, GPT-4, OpenAI released GPT-3 in June 2020. Significantly larger than GPT-2 with 175 billion parameters, GPT-3 was trained to not only generate higher quality content but also to finally have multilingual capabilities.
By opening up the world to the potential of AI, GPT models became game-changers in the field. OpenAI pushed plenty of companies to eventually create their own models, and now, more models that are even more accessible to people around the world are being created. The vast amounts of LLMs existing today have also revolutionized industries outside tech, like healthcare, finance, and customer service.
Very recently, OpenAI launched ChatGPT Enterprise, a ChatGPT tier specifically made for businesses, which allows businesses to access GPT-4 without usage caps with a performance that’s up to two times faster than previous versions. Clients can also input company data to train and customize.
What the Future Holds
With LLM technology already advancing quite swiftly in only a relatively short amount of time, there is definitely no telling how LLMs can become even more of a trailblazer in the coming years.
There is no doubt that this incredibly exciting technology will only become increasingly significant, not just for research in artificial intelligence but also for use cases across numerous industries.
Despite the challenges many enterprises have faced when dealing with LLMs, either in training or maintaining their performance quality, the tech’s future seems promising. Their ability to revolutionize the way humans interact with computers ought to be one researchers don’t take for granted and instead further utilize for innovation.

Meme & AI-Generated Picture




Job Posting
Chainalysis - Application Security Engineer - New York City, NY (Remote)
CrunchyRoll - Software Engineer III, Subscriptions - Culver City (Remote/Hybrid)
McDonald’s Global Technology - Sr SOC Manager - Chicago, IL (Remote)
ZocDoc- Business Systems Manager - New York City, NY (Remote/Hybrid)
Promote your product/service to Digger Insights’ Community
Advertise with Digger Insights. Digger Insights’ Miners are professionals and business owners with diverse Industry backgrounds who are looking for interesting and helpful tools, products, services, jobs, events, apps, and books. Email us [email protected]
Gives us feedback at [email protected]
Reply